study led by an Indian-origin researcher has used artificial intelligence
A recent study led by an Indian-origin researcher has used artificial intelligence (AI) tools to identify five subtypes of heart failure that could potentially help predict future risks for individual patients. Heart failure refers to the heart’s inability to effectively pump blood throughout the body. The current classification methods for heart failure do not accurately predict the progression of the disease.
The research, published in Lancet Digital Health, analyzed anonymized patient data from over 300,000 individuals aged 30 years and older who were diagnosed with heart failure in the UK over a 20-year period. Through various machine learning techniques, the study identified five subtypes: early onset, late onset, atrial fibrillation-related, metabolic (linked to obesity but with a low rate of cardiovascular disease), and cardiometabolic (linked to obesity and cardiovascular disease).
The study found differences in the risk of mortality within one year of diagnosis among the subtypes. The one-year all-cause mortality risks were as follows: early onset (20%), late-onset (46%), atrial fibrillation-related (61%), metabolic (11%), and cardiometabolic (37%).
To assist clinicians in determining the subtype of heart failure a patient has, the research team developed an app that could potentially improve predictions of future risk and facilitate discussions with patients.
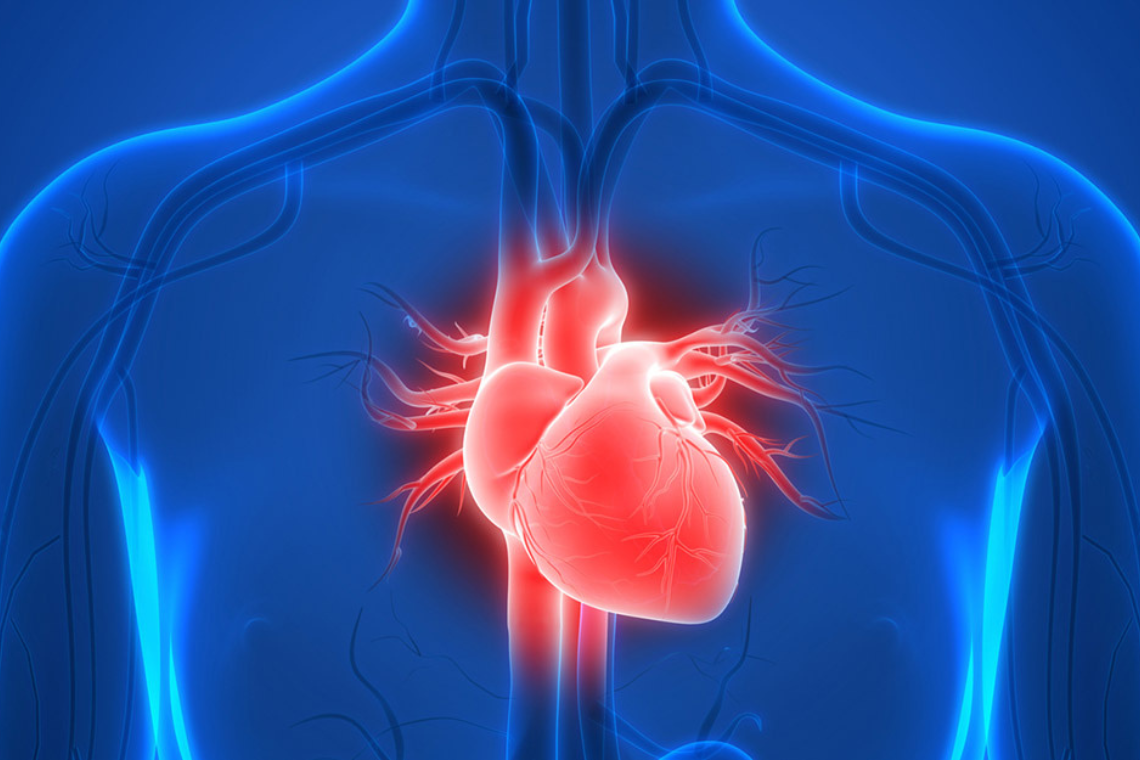
Lead author Professor Amitava Banerjee from UCL’s Institute of Health Informatics explained that the aim of the study was to enhance heart failure classification in order to better understand the disease’s course and communicate this information to patients. The team hopes that improved distinctions between heart failure subtypes will lead to more targeted treatments and prompt new perspectives on potential therapies.
The next phase of the research is to assess the practical implications for patients, including whether the classification method improves risk predictions, enhances the quality of information provided by clinicians, and impacts treatment decisions. Cost-effectiveness is another aspect that needs to be evaluated, and while the app designed in the study requires further clinical trials or research, it has the potential to assist in routine care.